Unlocking Opportunities in Self-Driving Cars: Training Data Essentials
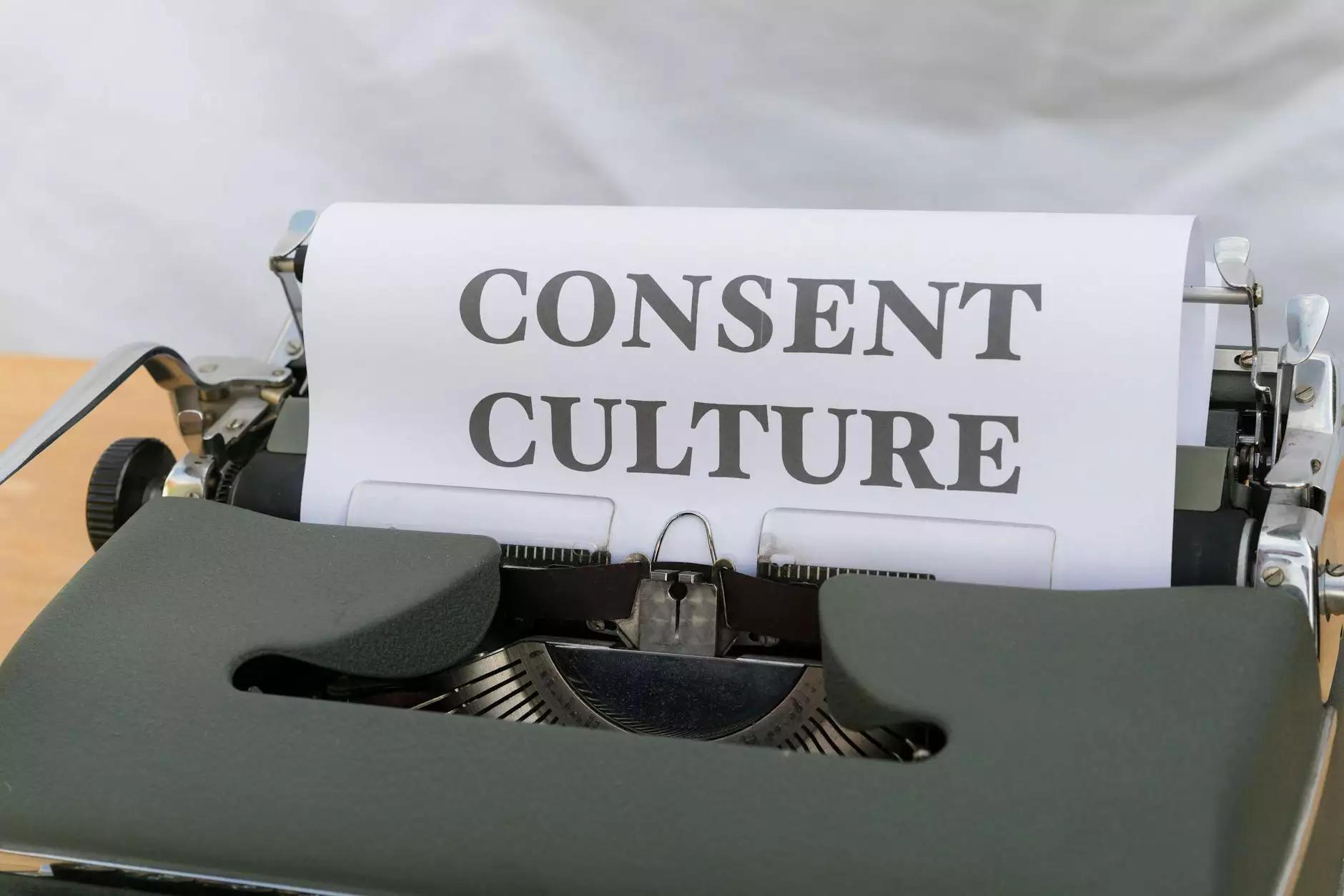
In the rapidly evolving world of technology, self-driving cars stand at the forefront, promising to revolutionize the way we perceive transportation. However, at the heart of this innovation lies a critical component: training data for self-driving cars. Understanding the significance of this data is vital not just for automotive companies, but also for a wide array of businesses looking to harness the potential of AI and machine learning.
The Role of Training Data in Autonomous Vehicles
Training data acts as the foundation upon which the machine learning algorithms of self-driving cars build their intelligence. This data is utilized to train AI systems to recognize and respond to a multitude of driving scenarios. The more diverse and extensive the training data, the more capable the system becomes at making decisions in real-time situations.
Types of Data Used in Training
Training data for self-driving cars can be categorized into several types:
- Visual Data: Images and videos captured by cameras installed in vehicles that provide visual context to the car’s surroundings.
- Lidar Data: Information gathered from Lidar sensors, which create precise 3D maps of the vehicle's environment.
- Radar Data: Data from radar sensors that help detect the speed and range of nearby objects, complementing visual data.
- GPS Data: Location data that assists in providing navigation and situational awareness for the vehicles.
- Annotated Data: Data that has been tagged and labeled to help the AI recognize different objects like pedestrians, signs, and other vehicles.
Why Quality Matters in Training Data
The quality of training data for self-driving cars is just as important as the quantity. High-quality data ensures that the AI can learn effectively, minimizing errors and improving decision-making capabilities. Here are several factors contributing to data quality:
- Diversity: A diverse dataset captures various driving conditions, weather patterns, and obstacles, making the self-driving system robust.
- Accuracy: Clear and correct labeling of data ensures that the AI can make accurate identifications and predictions.
- Completeness: Data should cover all potential scenarios a self-driving car may encounter to anticipate and react appropriately.
Real-World Scenarios and Challenges
Consider a scenario where a self-driving car encounters a child chasing a ball into the street. Training the AI to recognize this nuanced behavior requires a vast and well-annotated dataset that includes similar situations. The car must learn to understand not just the image of a child or a ball, but the context of the action—anticipating movement and potential danger.
The Impact of Sensor Technology on Data Collection
The evolution of sensor technology has fundamentally transformed how data for self-driving cars is collected. Advanced sensors like Lidar and radar, combined with high-resolution cameras, allow for comprehensive data acquisition. The synergy between these sensors results in:
- Improved Accuracy: Sensors complement each other to provide clearer and more accurate data regarding the environment.
- Enhanced Object Detection: Multiple sensor inputs enable better detection and classification of objects, even in challenging conditions.
- High-Fidelity Data Capture: The combination of various technologies leads to rich datasets that improve the training process.
Harvesting Data from Real Operations
Many companies deploy fleets of self-driving cars in real-world conditions to gather operational data. This practice not only captures a near-infinite variety of driving scenarios but also allows engineers to observe how vehicles perform in actual environments. Such data can then be fed back into the model for further training and refinement.
Ethics and Privacy Considerations in Data Collection
As we discuss the significance of training data for self-driving cars, it’s crucial to address the ethical implications surrounding data collection. Automakers must navigate various privacy concerns, especially when collecting data in populated areas. Here are key ethical considerations:
- Consent: Ensuring that data collection practices comply with privacy regulations and that users are informed.
- Anonymization: Implementing techniques to anonymize data to protect individuals’ identities during data analysis.
- Transparency: Being open about what data is collected and how it is used to foster trust among consumers.
The Future of Training Data in Autonomous Vehicles
As the technology continues to advance, the landscape of training data for self-driving cars is also evolving. Here are some future trends that are likely to shape the industry:
Integration of Synthetic Data
Synthetic data is generated using computer simulations to create scenarios that are hard to capture in the real world. This practice helps supplement traditional dataset collection by:
- Enhancing Diversity: Creating more varied scenarios that may not typically occur in real-world driving.
- Cost-Effectiveness: Reducing the need for extensive real-world data collection, which can be time-consuming and expensive.
Advancements in Machine Learning Techniques
As machine learning algorithms improve, they will become more adept at learning from smaller and more efficient data sets. This can lead to:
- Faster Learning Cycles: The AI could potentially learn to adapt to new scenarios quickly with minimal data.
- Personalized Learning: Systems might tailor their driving strategies based on user preferences and behaviors over time.
Conclusion: The Key to Unlocking the Future
In conclusion, training data for self-driving cars is not just a technical necessity; it symbolizes the future of how we will interact with transportation. As businesses like Keymakr.com continue to explore the implications of AI and autonomous technologies in home services, understanding the crucial dynamics of data utilization will be key to success. The road ahead is filled with potential, but it is founded upon the quality, accuracy, and ethical handling of the data we collect today. Let’s embrace this exciting journey and unlock the future of mobility!
training data for self driving cars